Machine Learning in Bioinformatics: Applications and Challenges
- Journal Series
- Jul 3, 2023
- 3 min read
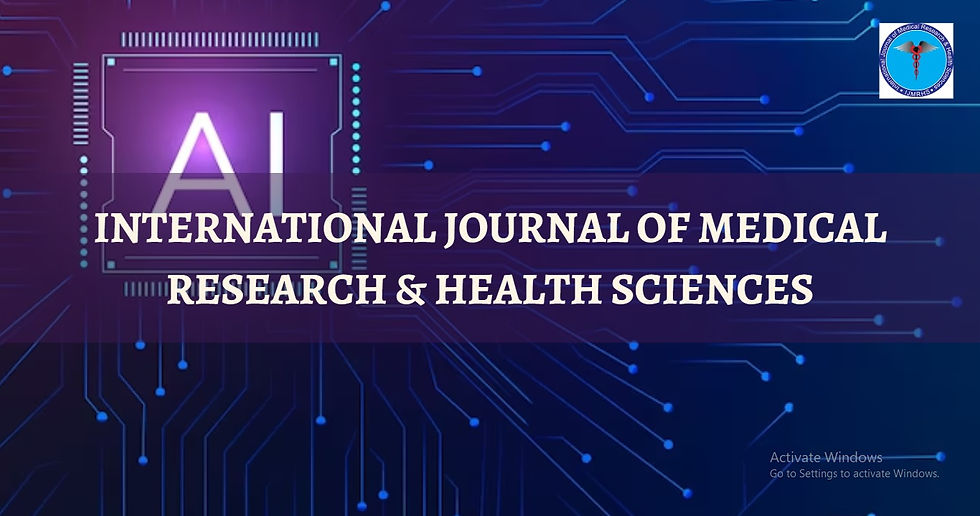
Introduction
In the sphere of bioinformatics, wherein full-size quantities of organic statistics are generated and analyzed, system learning has emerged as a powerful device for extracting meaningful insights. This weblog post aims to highlight the importance and significance of device getting to know in bioinformatics, provide an outline of bioinformatics and gadget mastering, explore the diverse packages of systems studying in bioinformatics, discuss popular machine learning algorithms used in this subject, and shed light on destiny directions and studies possibilities. Scholars and researchers from pinnacle universities globally, in particular the ones inquisitive about bioinformatics and system learning, will find this weblog put up informative. The statistics offered right here attract from the modern studies findings in Bioinformatics Journals.
Introduction to Bioinformatics and Machine Learning
Bioinformatics is an interdisciplinary discipline that combines biology, computer technological know-how, and records to investigate and interpret biological information. Key factors to consider include:
Biological facts: The sizable amounts of records generated from diverse biological experiments, such as genomics, proteomics, and metabolomics, require advanced computational strategies for evaluation.
Machine mastering: Machine learning algorithms, a subset of synthetic intelligence, offer the capacity to discover patterns and relationships in organic facts, main to novel insights and discoveries.
Applications of Machine Learning in Bioinformatics
Machine studying has discovered several applications in bioinformatics, revolutionizing the sector. Notable applications consist of:
Genomic sequence evaluation: Machine studying algorithms can are expecting gene characteristics, become aware of regulatory factors, and classify DNA sequences.
Protein shape prediction: Machine getting-to-know techniques allow the prediction of protein tertiary structures, facilitating drug layout and expertise protein capabilities.
Disease diagnosis and prognosis: Machine studying fashions can analyze patient facts, inclusive of genomic and clinical information, to aid in disease prognosis, diagnosis, and treatment selection.
Machine Learning Algorithms in Bioinformatics
Various gadgets gaining knowledge of algorithms have been tailored for bioinformatics evaluation. Important algorithms and strategies include:
Support Vector Machines (SVM): SVMs are powerful classifiers used for duties such as protein type and gene expression analysis.
Random Forest: Random Forest models excel in feature choice and classification obligations, making them treasured for figuring out disorder-related genetic markers.
Deep Learning: Deep studying algorithms, especially Convolutional Neural Networks (CNNs), have shown success in photograph evaluation, together with histopathology picture type and cell imaging.
Future Directions and Research Opportunities
The integration of system learning and bioinformatics keeps opening new avenues for research. Promising areas for future exploration consist of:
Multi-omics integration: Developing gadget learning methods to combine data from a couple of omics ranges, together with genomics, transcriptomics, and proteomics, to advantage of a complete knowledge of organic systems.
Explainable AI: Enhancing interpretability and transparency of device studying fashions in bioinformatics, permitting researchers to recognize the rationale at the back of predictions and discoveries.
Personalized medication: Leveraging gadget mastering to allow customized treatment strategies based on individual affected person traits, improving affected person outcomes.
In the end, machine learning to know has emerged as a powerful device in bioinformatics, enabling researchers to make full-size strides in information biological structures, sickness analysis, and remedy. By leveraging device-mastering algorithms and strategies, pupils and researchers from pinnacle universities internationally can release the capacity of great organic datasets. The integration of bioinformatics and device learning offers exciting potential for future studies and innovation. Staying up to date with the modern-day findings in Bioinformatics Journals empowers scholars to contribute to this hastily evolving discipline and make meaningful advancements in bioinformatics studies.
If you want to learn more about cell biology journals, then click here.
コメント